Central Limit Theorem Calculator
Enter the population mean, population standard deviation, and sample size to calculate the sample mean and standard deviation using the central limit theorem (CLT).
Results:
Sample Mean (μx̄)
Sample Standard Deviation (σx̄)
Steps to Solve Using the Central Limit Theorem
Find the Sample Mean
Provided the sample is sufficiently large, the sample mean will be equal to the population mean
μx̄ = μ
Find the Sample Standard Deviation
The central limit theorem provides the following formula to solve the standard deviation for a sample
σx̄ = σ / √n
On this page:
How to Use the Central Limit Theorem
The central limit theorem for sample means states that as you take larger samples of independent random variables and calculate their means, the sample means form their own normal distribution, which is known as the sampling distribution of the mean. This distribution has the same mean as the original distribution and a variance equaling the original variance divided by the sample size.
Given a known population mean value and a sufficiently large sample, the central limit theorem dictates that the sample mean will equal the population mean. The sample size that is big enough to provide these guarantees depends on context, however in practice rules of thumb about what is good enough can be surprisingly small, for example with some sources proposing samples of 100, 60, 30, or even in some cases as few as 12.[1]
Again, what approximation is good enough depends on context, but the notion that a sample of 30 or 60 could be sufficient is often surprising to those who have been taught to distrust surveys with fewer than 1,000 respondents.
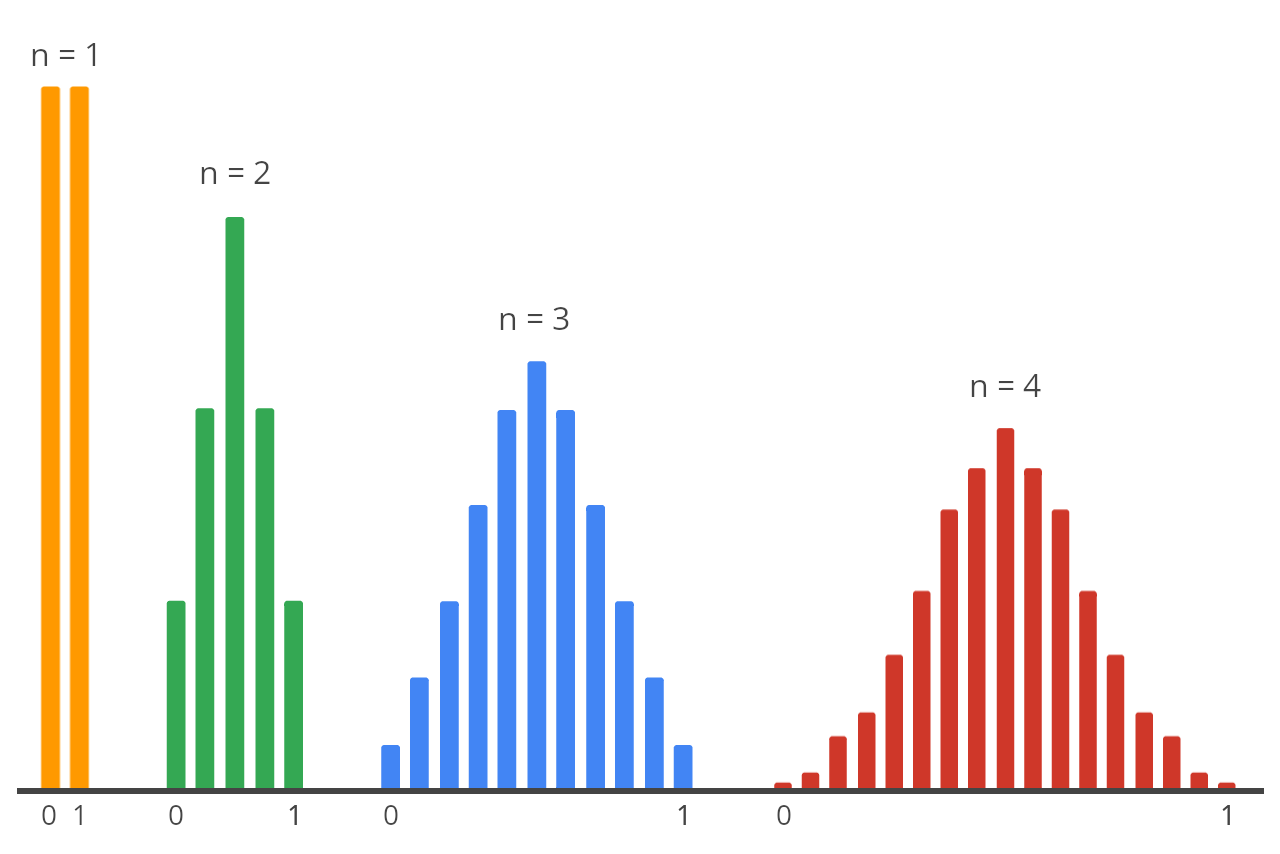
As you can see in the image above, as the sample size n increases, the values of each sample continue to be distributed around an equal mean.
Central Limit Theorem Formula
Using the central limit theorem, you can calculate the mean, standard deviation, and z-score given a sufficiently large sample size with the following formulas.
Remember:
mean: The average of the data set.
standard deviation: The measure of how spread out the values of a dataset are from the mean.
z-score: The measure that quantifies how many standard deviations a specific data point is away from the mean of a distribution.
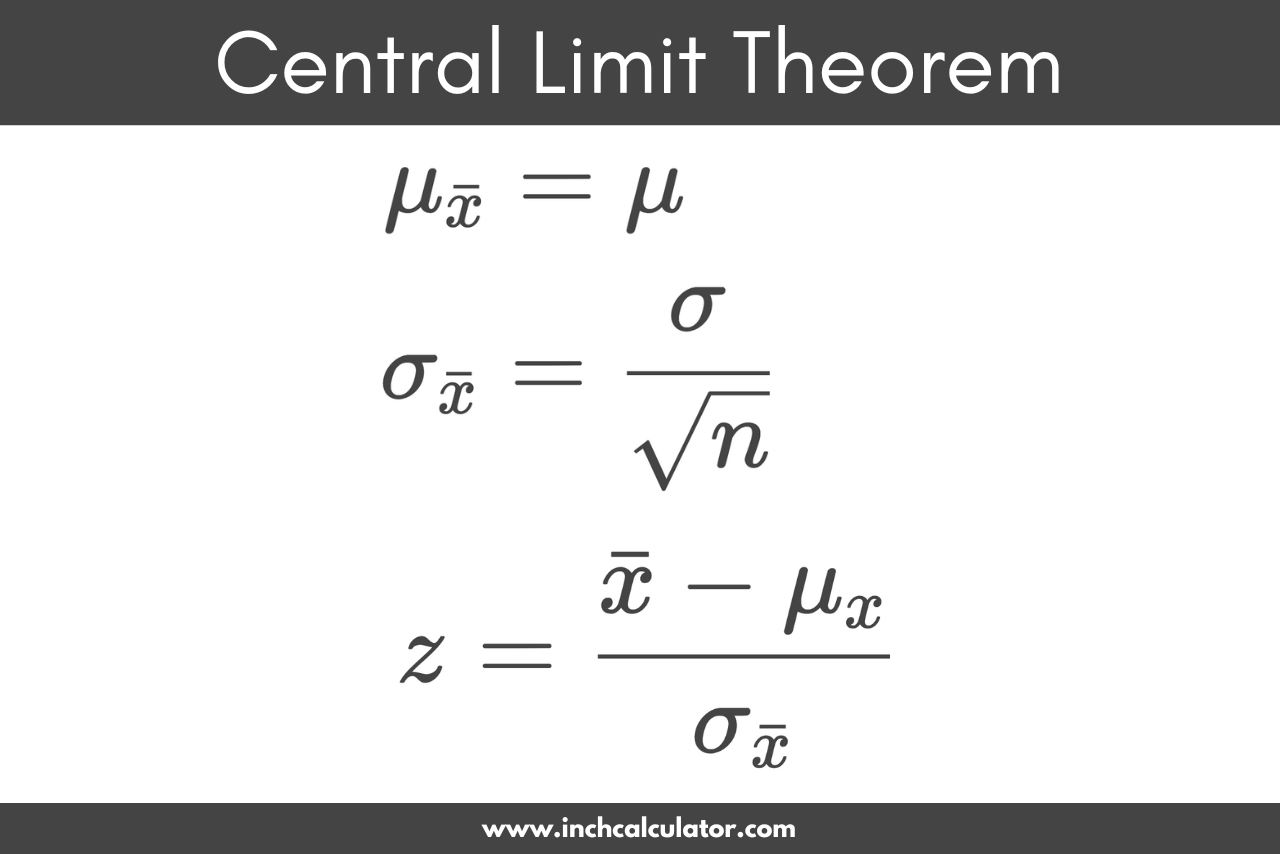
Let’s dig into each of these in a little more detail.
Sample Mean Formula
You can use the central limit theorem to find the sample mean. If μ is the mean of the population values x, then μx̄ is the mean of the sample values x̄.
The sample mean μx̄ is equal to the population mean μ.
μx̄ = μ
For example, if the population mean μ is equal to 50, then a sample size of 100 will have a mean μx̄ equal to 50 as well.
You can use our mean calculator to find the population mean μ.
Sample Standard Deviation Formula
You can also use the central limit theorem to find the standard deviation of a sample σx̄, given a known standard deviation of the population σ.
The central limit theorem provides the following formula to calculate the standard deviation of a sample.[2]
σx̄ = σ / √n
Thus, the central limit theorem states that the standard deviation of a sample σx̄ is equal to the standard deviation of the population σ divided by the square root of the sample size n.
This is also referred to as the standard error of the mean.
For example, if the population standard deviation σ is equal to 2, let’s find the standard deviation σx̄ of a sample with a size of 100.
σx̄ = 2 / √100
σx̄ = 2 / 10
σx̄ = 0.2
So, this sample will have a standard deviation σx̄ equal to 0.2.
You can use our standard deviation calculator to find the population standard deviation σ.
How to Find Probabilities Using the Central Limit Theorem
The central limit theorem can also be used to find the probabilities of sample means. Start by using the following formula to find the z-score.
z = x̄ – μx / σx̄
The z-score z is equal to the sample mean x̄ minus μ, which is the average of x and x̄, divided by the sample standard deviation σx̄.
Using the z-score, you can look up the probability of getting this mean using a z table or through the normal cumulative distribution function.
By applying a margin of error and confidence interval, we can make estimates on an independent random variable given a data set with a sufficiently large sample size and assess how good those estimates are.
References
- McLeod, S., What is central limit theorem in statistics?, Simply Psychology, 11/25/2019, https://www.simplypsychology.org/central-limit-theorem.html
- Illowsky, B., & Dean, S., The Central Limit Theorem for Sample Means (Averages), LibreTexts, 11/5/2021, https://stats.libretexts.org/@go/page/757